Publicaciones científicas
An explainable machine learning approach for hospital emergency department visits forecasting using continuous training and multi-model regression
C Peláez-Rodríguez 1 , R Torres-López 2 , J Pérez-Aracil 2 , N López-Laguna 3 , S Sánchez-Rodríguez 4 , S Salcedo-Sanz 2
Background and objective: In the last years, the Emergency Department (ED) has become an important source of admissions for hospitals. Since late 90s, the number of ED visits has been steadily increasing, and since Covid19 pandemic this trend has been much stronger. Accurate prediction of ED visits, even for moderate forecasting time-horizons, can definitively improve operational efficiency, quality of care, and patient outcomes in hospitals.
Methods: In this paper we propose two different interpretable approaches, based on Machine Learning algorithms, to accurately forecast hospital emergency visits. The proposed approaches involve a first step of data segmentation based on two different criteria, depending on the approach considered: first, a threshold-based strategy is adopted, where data is divided depending on the value of specific predictor variables. In a second approach, a cluster-based ensemble learning is proposed, in such a way that a clustering algorithm is applied to the training dataset, and ML models are then trained for each cluster.
Results: The two proposed methodologies have been evaluated in real data from two hospital ED visits datasets in Spain. We have shown that the proposed approaches are able to obtain accurate ED visits forecasting, in short-term and also long-term prediction time-horizons up to one week, improving the efficiency of alternative prediction methods for this problem.
Conclusions: The proposed forecasting approaches have a strong emphasis on providing explainability to the problem. An analysis on which variables govern the problem and are pivotal for obtaining accurate predictions is finally carried out and included in the discussion of the paper.
CITA DEL ARTÍCULO Comput Methods Programs Biomed. 2024 Jan 23:245:108033. doi: 10.1016/j.cmpb.2024.108033
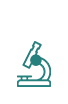